TransGlow: Attention-augmented Transduction model based on Graph Neural Networks for Water Flow Forecasting
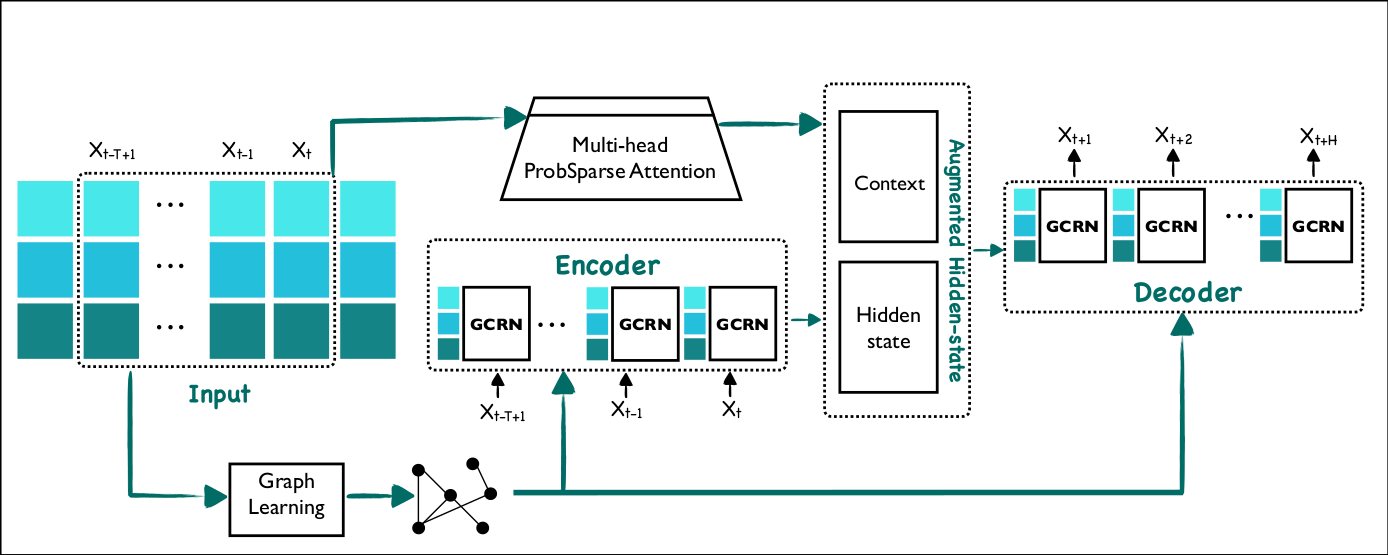
The paper "TransGlow: Attention-augmented Transduction model based on Graph Neural Networks for Water Flow Forecasting" by Naghmeh Shafiee Roudbari, Charalambos Poullis, Zachary Patterson, and Ursula Eicker, has been accepted for publication in the International Conference on Machine Learning and Applications (ICMLA), 2023.
TL;DR: The hydrometric prediction of water quantity is useful for a variety of applications, including water management, flood forecasting, and flood control. However, the task is difficult due to the dynamic nature and limited data of water systems. Highly interconnected water systems can significantly affect hydrometric forecasting. Consequently, it is crucial to develop models that represent the relationships between other system components. In recent years, numerous hydrological applications have been studied, including streamflow prediction, flood forecasting, and water quality prediction. Existing methods are unable to model the influence of adjacent regions between pairs of variables. In this paper, we propose a spatiotemporal forecasting model that augments the hidden state in Graph Convolution Recurrent Neural Network (GCRN) encoder-decoder using an efficient version of the attention mechanism.