Unsupervised Structure-Consistent Image-to-Image Translation
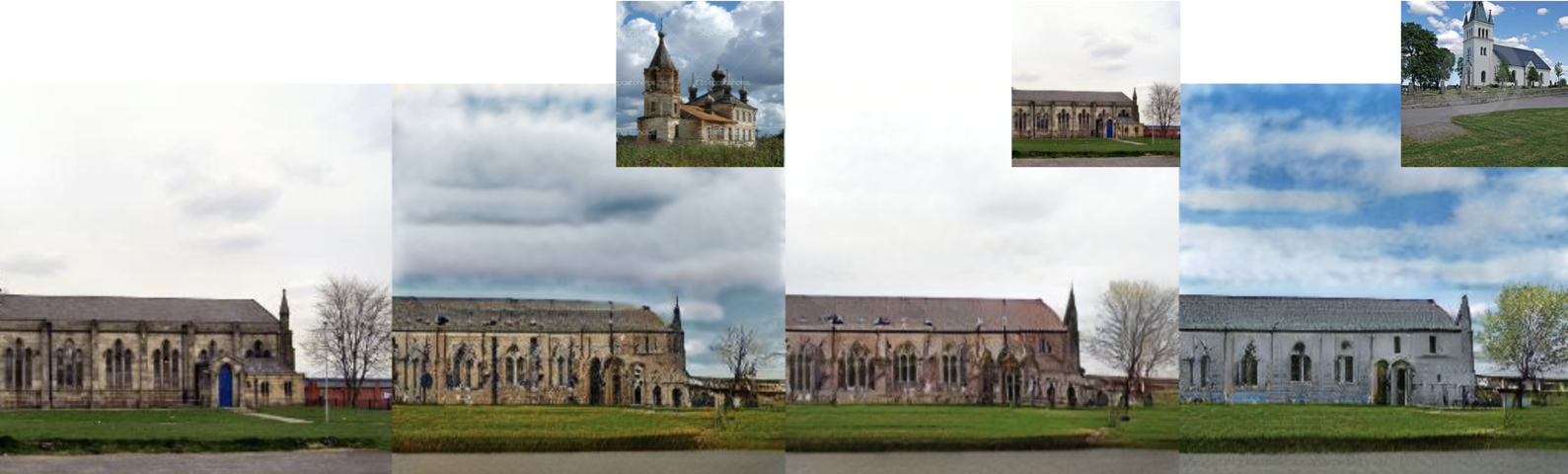
The preprint of our paper Unsupervised Structure-Consistent Image-to-Image Translation is available on arxiv.org. The work is co-authored by Shima Shahfar and Charalambos Poullis.
TL;DR: We present a structure-consistent image-to-image translation technique based on the swapping autoencoder. We introduce an auxiliary module which forces the generator to learn to reconstruct an image with an all-zero texture code, encouraging better disentanglement between the structure and texture information and significantly reducing training time. Our method works on complex domains such as satellite images where state-of-the-art are known to fail.
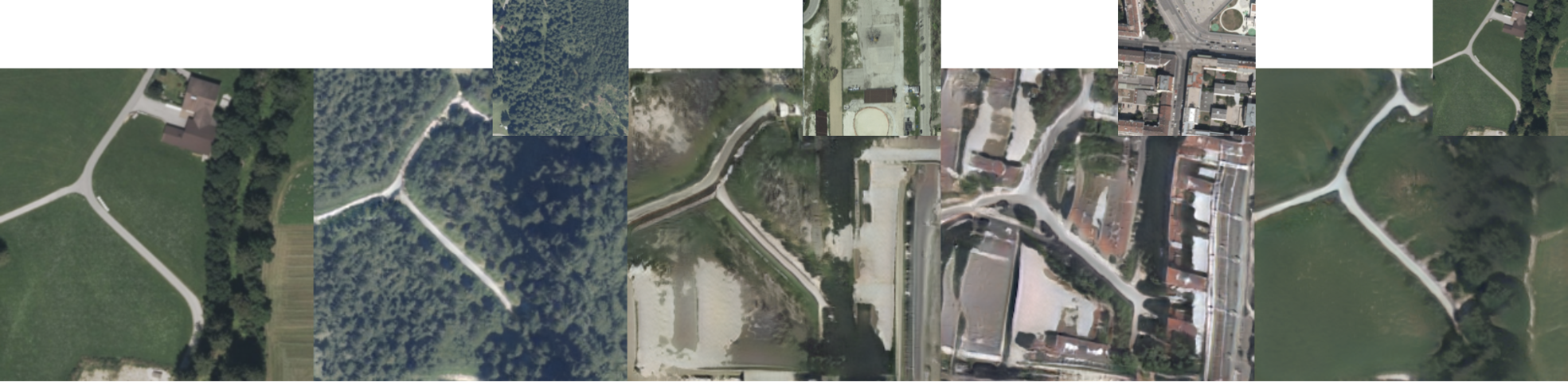
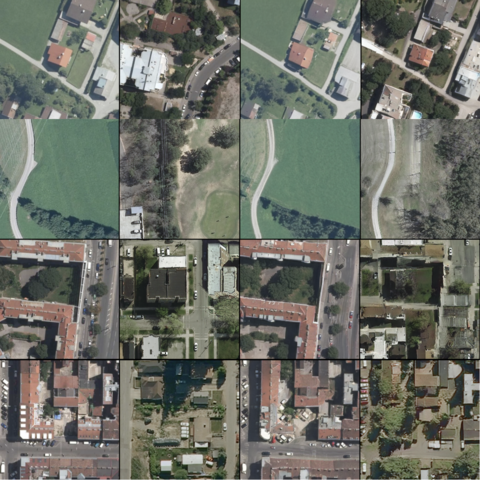
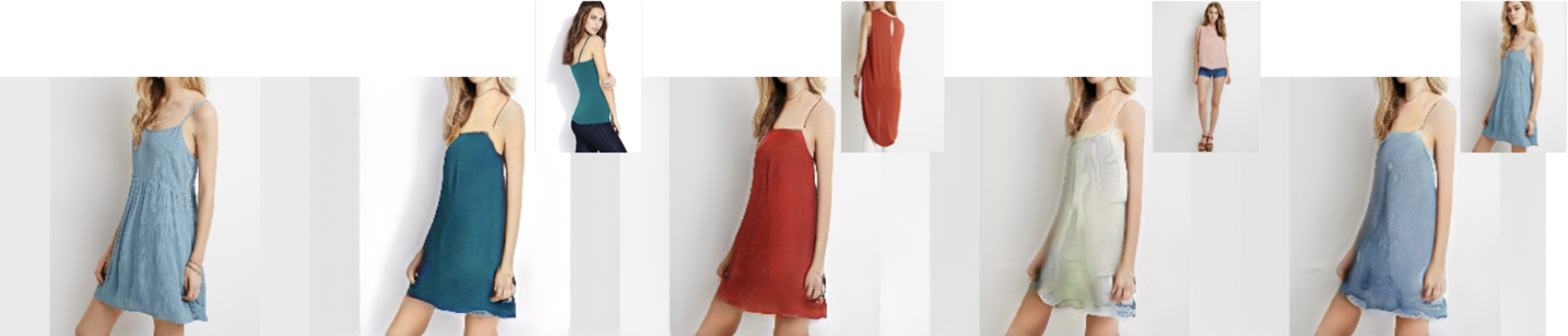
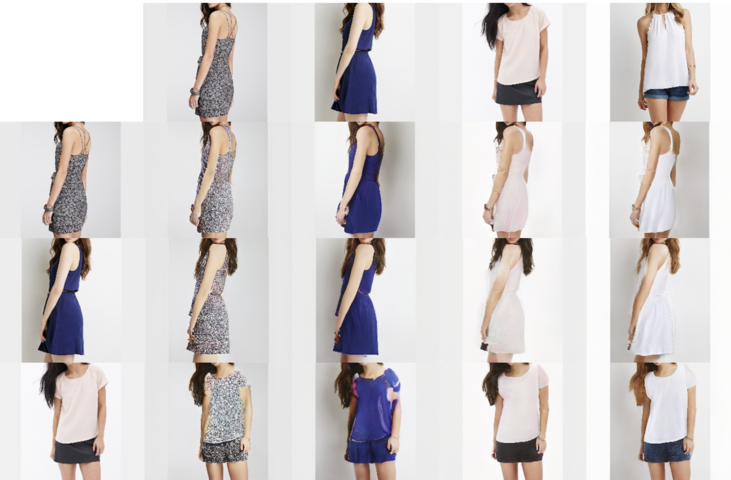
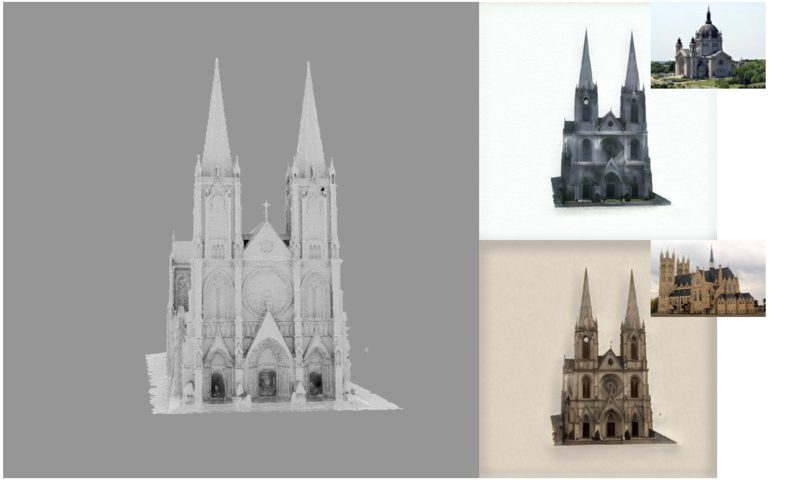